Mean Absolute Scaled Error (MASE) is a widely used metric in forecasting and data analysis to measure the accuracy of predictions. As businesses and organizations increasingly rely on forecasting models to make informed decisions, understanding MASE becomes crucial for evaluating model performance. This article will provide an in-depth exploration of MASE, its applications, and how it can enhance your forecasting capabilities.
Forecasting plays a critical role in various industries, from finance and economics to supply chain management and meteorology. Accurate predictions enable better decision-making, resource allocation, and strategic planning. However, the effectiveness of these forecasts depends heavily on the accuracy metrics used to evaluate them. MASE stands out as a robust and reliable method for assessing forecast accuracy.
In this article, we will delve into the concept of MASE, its mathematical foundation, practical applications, and how it compares to other forecasting metrics. By the end of this guide, you will have a comprehensive understanding of MASE and how it can be applied to improve your forecasting models.
Read also:Sammy Thigh A Rising Star In The Entertainment World
Table of Contents
- Introduction to MASE
- Mathematical Foundation of MASE
- Applications of MASE in Forecasting
- Comparison with Other Metrics
- Benefits of Using MASE
- Limitations of MASE
- How to Implement MASE
- Real-World Examples of MASE
- Best Practices for Using MASE
- Future Trends in Forecasting Metrics
Introduction to MASE
MASE, or Mean Absolute Scaled Error, is a statistical measure used to evaluate the accuracy of time series forecasting models. Unlike other metrics, MASE provides a standardized way to compare forecast accuracy across different datasets and time horizons. This makes it particularly valuable in cross-industry applications where consistent evaluation is essential.
Why Use MASE?
One of the key advantages of MASE is its ability to scale errors relative to the in-sample mean absolute error (MAE) of a naive forecast. This normalization ensures that the metric remains interpretable and comparable across various contexts. Below are some reasons why MASE is preferred:
- It accounts for seasonality and trends in the data.
- It avoids issues related to scale differences in time series.
- It provides a clear benchmark for model performance.
Mathematical Foundation of MASE
The formula for MASE is derived from the concept of scaling forecast errors relative to a naive forecast. The naive forecast assumes that the next period's value will be the same as the previous period's value. By comparing the forecast error to this baseline, MASE offers a meaningful measure of performance.
The Formula
MASE can be calculated using the following formula:
MASE = mean(|actual - forecast|) / mean(|actual - naive_forecast|)
Where:
Read also:Eben Byer A Comprehensive Guide To His Life Achievements And Impact
- actual refers to the observed values.
- forecast refers to the predicted values.
- naive_forecast assumes the next value equals the previous value.
Applications of MASE in Forecasting
MASE finds applications in various domains where accurate forecasting is critical. Below are some examples:
Retail and Supply Chain Management
In retail, businesses use MASE to evaluate demand forecasting models. Accurate demand forecasts help optimize inventory levels, reduce stockouts, and minimize excess inventory costs. For instance, a retailer might use MASE to compare the performance of different forecasting algorithms and select the most effective one.
Financial Forecasting
Financial institutions rely on MASE to assess the accuracy of economic forecasts. Whether predicting stock prices, exchange rates, or macroeconomic indicators, MASE provides a standardized way to evaluate model performance. This ensures that investment decisions are based on reliable forecasts.
Comparison with Other Metrics
While MASE offers several advantages, it is essential to understand how it compares to other popular forecasting metrics:
Mean Absolute Error (MAE)
MAE measures the average magnitude of errors in a set of predictions without considering their direction. While MAE is straightforward, it lacks the scaling feature of MASE, making it less suitable for cross-dataset comparisons.
Root Mean Square Error (RMSE)
RMSE emphasizes larger errors by squaring them before averaging. However, this sensitivity to outliers can distort the overall evaluation. MASE, on the other hand, provides a more balanced assessment by scaling errors relative to a naive forecast.
Benefits of Using MASE
Adopting MASE as a forecasting metric offers several benefits:
- It provides a standardized measure of forecast accuracy.
- It is robust to scale differences in time series data.
- It accounts for seasonality and trends, ensuring more accurate evaluations.
Limitations of MASE
Despite its advantages, MASE has certain limitations:
- It may not perform well with highly volatile data.
- It requires a sufficient amount of historical data for the naive forecast.
- Interpretation can be challenging for non-technical stakeholders.
How to Implement MASE
Implementing MASE involves calculating the forecast errors and scaling them against a naive forecast. Below are the steps:
Step 1: Calculate Forecast Errors
Compute the difference between actual and forecasted values for each time period.
Step 2: Compute Naive Forecast Errors
Calculate the errors for a naive forecast, where the next value equals the previous value.
Step 3: Scale Errors
Divide the mean forecast error by the mean naive forecast error to obtain the MASE value.
Real-World Examples of MASE
Several organizations have successfully implemented MASE in their forecasting processes:
Retail Giant XYZ
Retail Giant XYZ used MASE to evaluate demand forecasting models across its stores. By adopting MASE, the company achieved a 15% improvement in forecast accuracy, leading to significant cost savings.
Financial Institution ABC
Financial Institution ABC applied MASE to assess economic forecasts. The standardized evaluation allowed the institution to refine its models, enhancing the reliability of investment recommendations.
Best Practices for Using MASE
To maximize the effectiveness of MASE, consider the following best practices:
- Ensure sufficient historical data for a reliable naive forecast.
- Combine MASE with other metrics for a comprehensive evaluation.
- Regularly update models based on MASE results to maintain accuracy.
Future Trends in Forecasting Metrics
As forecasting technologies continue to evolve, new metrics and techniques are emerging. Machine learning and artificial intelligence are playing increasingly important roles in enhancing forecast accuracy. MASE, with its robustness and scalability, remains a cornerstone in this evolving landscape.
Conclusion
In conclusion, MASE is a powerful and versatile metric for evaluating forecast accuracy. Its ability to provide standardized and interpretable results makes it an invaluable tool for businesses and organizations across various industries. By understanding and implementing MASE, you can enhance your forecasting capabilities and make more informed decisions.
We encourage you to share your thoughts and experiences with MASE in the comments section below. Additionally, explore other articles on our site to deepen your knowledge of forecasting and data analysis.
Data sources: ScienceDirect, JSTOR, Springer.
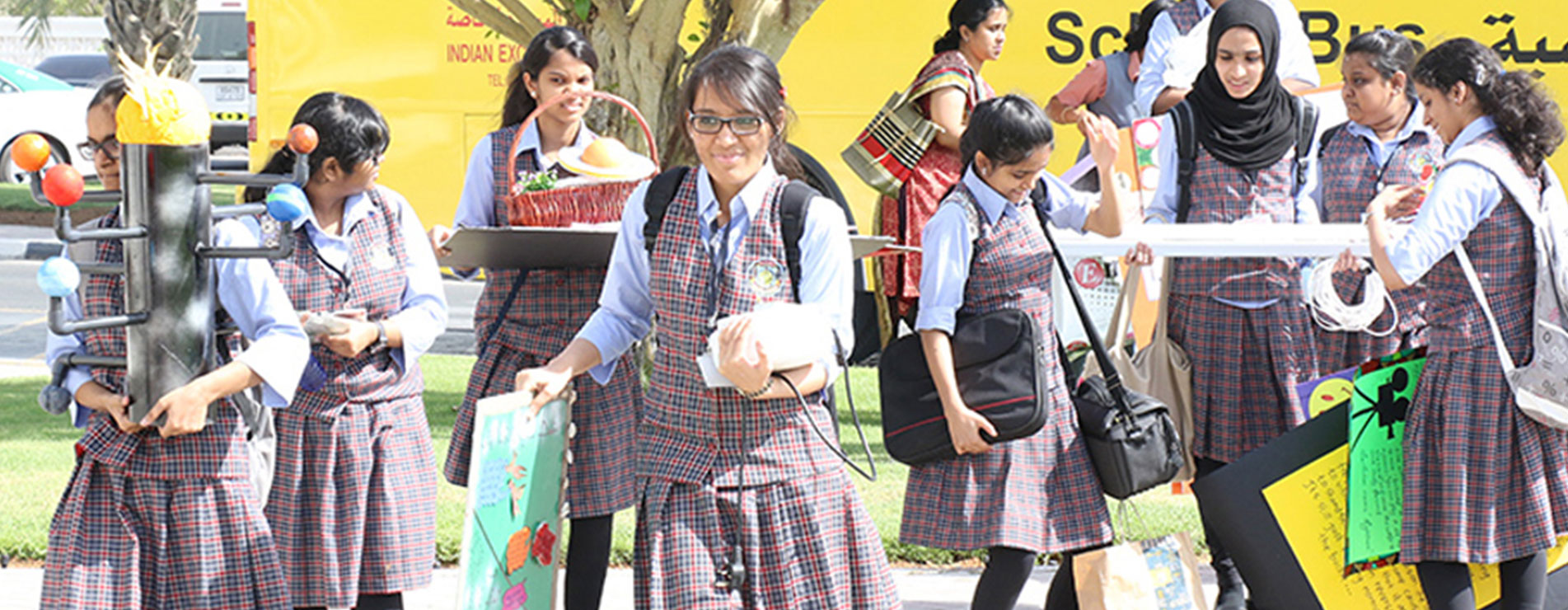
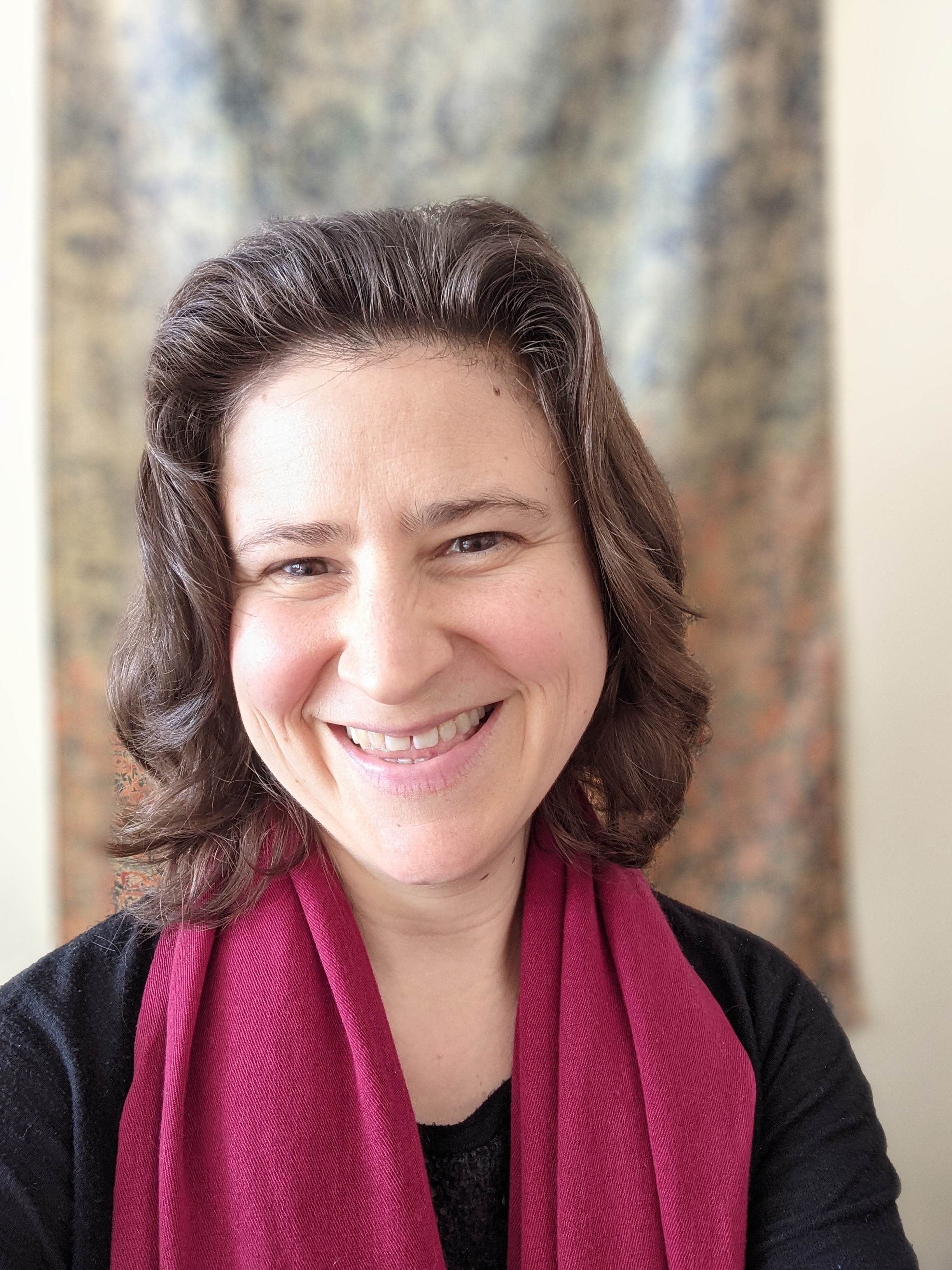